Role of Historical Data and Global Trends in Demand Estimation
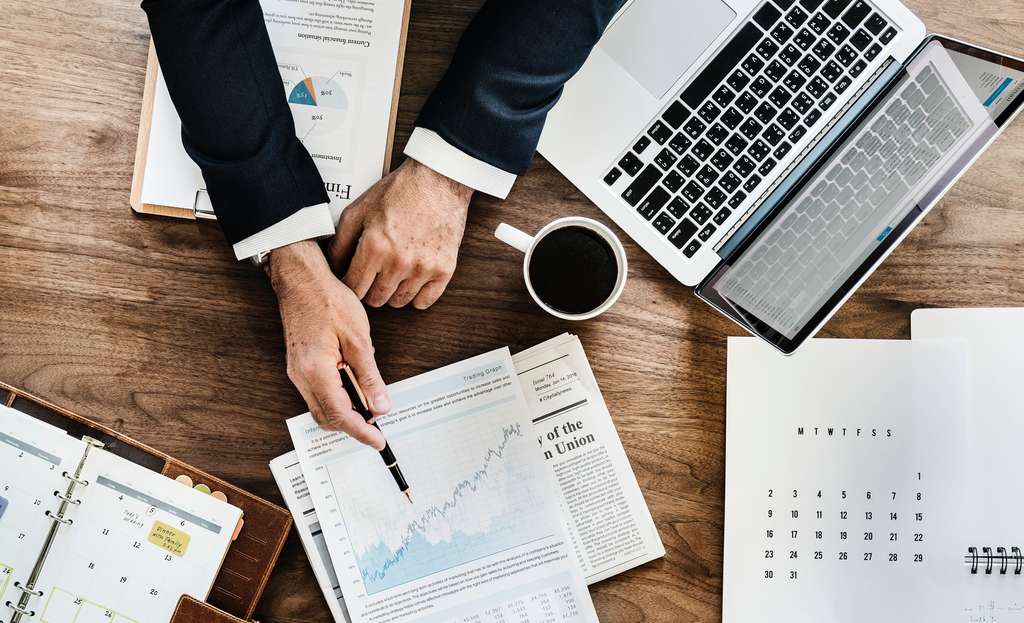
Historical Data shows previous patterns of demand and supply examples include sales records, customer orders, inventory levels, market share, pricing, promotions, seasonality, and outside variables. For your forecast, you must collect enough data to span an appropriate time range and frequency. For instance, you want at least 12 months of historical data in order to anticipate monthly demand for a whole year. Additionally, you must organise and clean up your data, eliminating any outliers, mistakes, or missing numbers that can affect your forecast.
Historical Data for Demand Forecasting
It is essential to comprehend the importance of demand forecasting. Manufacturers can effectively allocate resources, link inventory levels with expected demand, and optimise their production schedules. As a result, expenses are decreased, customer satisfaction is raised, decision-making is improved, and profitability is eventually boosted. However, it can be difficult for producers to estimate future demand with any degree of accuracy. These difficulties emphasise the necessity for reliable forecasting techniques that can deal with the changing nature of demand trends. In order to overcome these difficulties and increase demand forecasting accuracy, historical data is crucial. With the use of these insightful historical demand patterns, producers may spot trends, seasonality, and other demand-influencing elements.
Importance of Historical Data
Understanding previous demand patterns and behaviours requires using historical data as a point of reference. It gives manufacturers a foundation for recognising recurring patterns, seasonality, and changes in client demand.
Manufacturers may decide wisely regarding resource allocation, inventory control, and production levels by using historical data. A data-driven approach to demand forecasting is made possible by historical data, allowing manufacturers to increase forecast accuracy and streamline their processes.
Sales Data
One of the most basic categories of historical data utilised in predicting is this one. It contains data on sales volumes, income, and purchasing trends of customers over a certain time frame. Sales data helps discover any repeating trends or seasonality and sheds light on prior demand levels.
Customer Data
Customer data includes details on specific clients, including their purchasing patterns, interests, demographics, and behaviour. Additionally, segmenting their client base, understanding demand variances across various consumer categories, and customising their projections are all made possible by customer data analysis for manufacturers.
Identity Data
Name | Both first and last |
Mailing info | Physical address to which you can send packages or mail. |
Digital Contact info | This includes both the email address and the telephone number. If the customer has a preferred method, it should be noted. |
Social Media | This includes both the social media profiles that the customer uses on different platforms, as well as information about which platform they prefer. |
Personal Information | Birthday, account info related to your company. |
Descriptive Data
Professional details | The Current company name and their position within it. |
Family info | Marital Status |
General lifestyle | House, Car, Hobbies, etc. |
Quantitive Data
Data that can be measured is known as quantitative data. The majority of this data relates to consumer contacts with your business, whether they occur in person, over the phone, or on your website. Since this data always comes down to numbers, CRM metrics are where it is most frequently used.
The number of purchases that the customer has made with your company.The average value of their purchase history.The frequency of their visitations to your website.The average amount of time they spend on your website.How often do they engage with your company on social media? |
Qualitative Data
Comparatively, qualitative data tends to be more descriptive. Customers’ attitudes, motives, and behaviours that are related to purchase decisions are typically included. Since it is hard to quantify this information, businesses frequently employ surveys and feedback enquiries to obtain it directly from customers.
Market Data
Market data includes details regarding competitive performance, market trends, and general market circumstances. Data on market size, market share, price trends, and market dynamics are all included. Manufacturers may better grasp the external elements affecting demand and incorporate them into their forecasting models by analysing market data.
External Data Sources
Manufacturers can use external data sources in addition to internal data sources to improve the accuracy of their forecasts. This includes information from market research studies, government statistics, industry publications, social media trends, and other pertinent outside sources. Additional context and insights into market trends, consumer behaviour, and developing patterns are provided by external data.
Contribution of Historical Data
- Demand trends: Manufacturers can discover recurrent demand patterns like seasonality, cyclicality, or trend-based fluctuations with the aid of historical data. Manufacturers can modify their projections to take into account anticipated variations in demand by recognising these trends.
- Data-Driven Insights: Long-term patterns in demand may be found and analysed using historical data. Manufacturers benefit from its aid in comprehending changes in consumer preferences, market dynamics, and market trends. Manufacturers may modify their plans and foresee changes in future demand by recognising these tendencies.
- Evaluating forecast accuracy: The validity of demand forecast accuracy may be verified using historical data. Manufacturers may evaluate the accuracy of their forecasting models, pinpoint areas for development, and enhance their future predictions by contrasting the predicted values with the actual historical data.
- Analyse prior changes: Manufacturers can analyse prior demand changes and determine their reasons using historical data. Manufacturers can modify their forecasting models and create backup plans to lessen the effects of future changes by recognising the causes of demand volatility.
- Enhancing projections: Manufacturers may develop data-driven forecasts and increase the precision of their projections by incorporating previous data into demand forecasting algorithms. It offers a strong basis for comprehending historical demand patterns, seeing trends, and coming to wise conclusions to optimise operations and resource allocation.
Storage of Historical Data
- Historical data can be stored in a variety of ways, including databases, spreadsheets and text files.
- It is important to choose a storage method that is efficient and scalable so historical data can be easily accessed and analyzed.
- There are many options available for storing historical data.
- The best option for a particular organization depends on the specific needs and requirements.
- Storage capacities have increased significantly in recent years and cloud storage has taken much of the burden of storage administration from many enterprises.
- However, large organisations with strict compliance requirements and privacy controls also have options to store historical data in a private server or data centre.
Retention of Historical Data
Some organizations are required by law or regulation to retain historical data for a certain period of time. For example, businesses in the financial services industry are subject to Financial Industry Regulatory Authority (FINRA) rules, which require the retention of business records for varying lengths of time depending on the type of record. Other organizations may have internal policies for how long historical data should be retained. For example, a company may keep financial records for seven years or customer service records for five years.
Conclusion
It is important to consult with legal and compliance teams to determine how long historical data needs to be retained. Once a decision has been made, it is important to have a plan in for how historical data will be stored and managed over time. Because data storage requires resources to maintain, data lifecycle management (DLM) is recommended to ensure that data is not maintained without good reason or for longer than necessary and that it is properly archived or disposed of as appropriate.